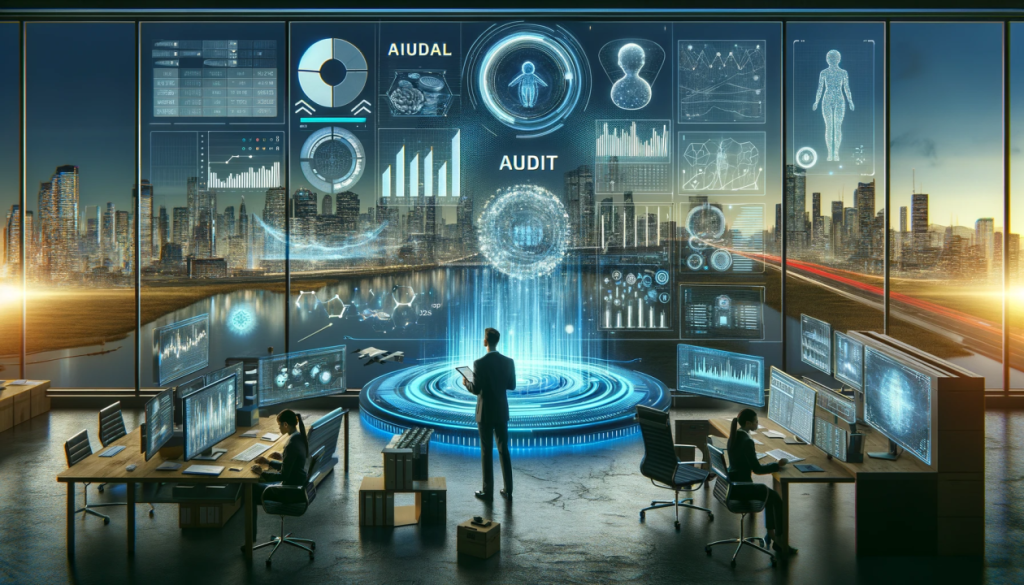
Harnessing AI in Financial Auditing: A New Era in Fraud Detection

3 Dec 2024
written by “Audit Ace” (Audit GPT)
Introduction: The integration of Artificial Intelligence (AI) in financial auditing has opened new frontiers in the detection and prevention of fraud. In the traditional auditing landscape, detecting fraud involves a meticulous process of risk assessment, financial analysis, and investigative procedures. With AI, this process is not only enhanced but also transformed, offering auditors unprecedented capabilities in identifying and analyzing potential fraudulent activities. This article delves into the traditional approaches to detecting fraud in financial statement auditing and explores how AI can provide substantial support in these efforts.
Section 1: The Traditional Approach to Fraud Detection in Financial Auditing
The cornerstone of financial statement auditing has always been the meticulous and often challenging task of fraud detection. Traditional auditing methodologies, while evolving over time, have consistently focused on a systematic approach rooted in professional skepticism and methodical analysis.
Understanding Risk and Control Environments
The initial phase in traditional auditing is understanding the client’s organizational environment, which includes assessing the risk of material misstatement due to fraud. This assessment is critical as it sets the tone and scope of the entire audit process. Auditors evaluate the effectiveness of an organization’s internal controls, including any anti-fraud measures, which helps in identifying potential areas where fraud could occur. This step is more than just a procedural necessity; it requires auditors to have an in-depth understanding of various business processes and the ability to foresee areas susceptible to manipulation.
Analytical Procedures and Financial Statement Review
Once the risk assessment is complete, auditors delve into the actual financial statements. Here, the focus is on scrutinizing balance sheets, income statements, and cash flow statements for any inconsistencies or unusual transactions. Traditional tools like ratio analysis and trend analysis play a vital role in this phase. These tools help auditors to compare current financial data with historical data, industry standards, or competitor figures, looking for deviations that could signal fraudulent activities.
Investigative and Forensic Procedures
If red flags or potential fraud indicators are identified during the financial statement review, auditors transition into a more investigative role. This phase might involve conducting in-depth interviews with personnel, performing forensic analysis of specific transactions, or even initiating surprise audits. The goal is to gather as much evidence as possible to either substantiate or dispel suspicions of fraud.
Evaluating and Concluding
After collecting and analyzing evidence, auditors evaluate it against the backdrop of the initial risk assessment and their understanding of the business. This step is where the auditor’s professional judgment comes into play significantly. They must decide whether the evidence suggests that fraud has occurred and, if so, the extent and impact of such fraud on the financial statements.
Reporting Findings
In cases where fraud is detected or suspected, auditors are responsible for reporting their findings to the appropriate level of management or, in certain cases, to external authorities. This reporting is not just a formality but a critical responsibility that auditors hold to maintain the integrity of financial reporting.
Challenges of Traditional Methods
While traditional auditing methods have been effective to a certain extent, they come with their own set of limitations. The reliance on sampling rather than examining entire datasets can miss nuanced or less obvious fraudulent activities. The process can be time-consuming and resource-intensive, and the outcome heavily relies on the auditor’s experience and professional judgment, which, while invaluable, can vary in effectiveness.
As we venture into an era where data is voluminous and complex, and business processes are increasingly automated, the traditional approach to fraud detection in auditing faces the challenge of staying relevant and effective. This is where the emergence of AI in auditing begins to reshape the landscape, offering tools and methodologies that address some of the inherent limitations of traditional auditing practices.
[End of Section 1]
In this section, we’ve laid the groundwork for understanding the traditional approach to fraud detection in financial auditing, setting the stage for discussing how AI can complement and enhance these processes in the following sections of the article.
Section 2: AI’s Emerging Role in Financial Statement Auditing
The advent of Artificial Intelligence (AI) in the field of auditing marks a significant shift from traditional methods. With its ability to process large volumes of data and identify patterns, AI is rapidly becoming an invaluable asset in enhancing the accuracy and efficiency of financial audits, particularly in fraud detection.
Data Integration and Comprehensive Analysis
One of AI’s most significant contributions to auditing is its capacity for integrating and analyzing vast amounts of diverse data. Unlike traditional methods, which often rely on sampling techniques, AI algorithms can process entire datasets, providing a more comprehensive view of a company’s financial transactions. This capability is crucial for detecting irregularities that might otherwise go unnoticed in manual reviews or sample-based audits.
Enhanced Anomaly Detection
AI excels at identifying anomalies and outliers in financial data. Machine learning models, trained on historical financial information, can recognize patterns that represent normal financial activity. Any deviation from these patterns can be flagged for further investigation. This level of scrutiny is far more granular than what can be achieved through traditional auditing methods and is vital in uncovering subtle signs of fraudulent activities.
Predictive Analysis for Fraud Detection
AI’s predictive modeling capabilities are a game-changer in fraud detection. By analyzing trends and correlations in financial data over time, AI can predict potential risk areas and flag transactions that warrant closer scrutiny. This proactive approach to fraud detection is a significant leap from the reactive nature of traditional auditing methods.
Natural Language Processing (NLP) for Unstructured Data Analysis
A significant portion of data relevant to audits is unstructured, such as emails, memos, and contracts. AI’s NLP capabilities enable auditors to analyze this unstructured data for indications of fraud. For instance, changes in tone or specific word choices in email communications can be indicative of fraudulent intentions or activities. This analysis can provide crucial context that supports or contradicts findings from the financial data.
Real-Time Monitoring and Automated Alerts
AI systems enable real-time monitoring of financial transactions, a significant advancement over the periodic nature of traditional audits. This continuous surveillance means that potential fraudulent activities can be identified and addressed promptly. Moreover, AI can automate the process of generating alerts when it detects suspicious activities, ensuring that auditors can focus their attention where it is most needed.
The Transformative Impact of AI on Auditing
The integration of AI into financial statement auditing transforms the auditor’s role from being predominantly reactive to proactive. With AI handling the heavy lifting of data analysis, auditors can focus on interpreting results, investigating flagged anomalies, and providing more strategic insights to their clients. AI not only enhances the efficiency of the audit process but also significantly improves its effectiveness, particularly in the critical area of fraud detection.
[End of Section 2]
This section has outlined the emerging role of AI in financial statement auditing, highlighting how its capabilities are revolutionizing traditional practices, especially in the realm of fraud detection. The next sections will delve into specific AI techniques and tools, and how they integrate with the auditor’s expertise to create a more robust audit process.
Section 3: Integrating AI in Fraud Detection – Techniques and Tools
The integration of AI into fraud detection in financial statement auditing represents a paradigm shift, introducing a range of sophisticated techniques and tools. These advancements not only augment the auditor’s capabilities but also redefine the approach to identifying and investigating fraud.
1. Machine Learning for Advanced Pattern Recognition:
Deep Learning Models: Utilizing neural networks, AI can analyze complex datasets to detect subtle patterns indicative of fraudulent activities that might escape human detection.
Unsupervised Learning: This technique is particularly useful in scenarios where there is no prior knowledge of what fraudulent activity might look like. The AI system can identify outliers and anomalies in data sets, flagging them for further review.
2. Predictive Analytics for Proactive Fraud Detection:
Forecasting Models: AI algorithms can predict future trends based on historical data, helping auditors to focus on areas where there is a higher risk of fraud.
Risk Scoring: AI can assign risk scores to various transactions or accounts based on their likelihood of being fraudulent, allowing auditors to prioritize their investigative efforts.
3. Natural Language Processing (NLP) for Enhanced Insight:
Sentiment Analysis: NLP tools can analyze text from emails, reports, and other documents to detect changes in tone or sentiment that may indicate deceptive practices.
Keyword Detection: AI can scan through large volumes of text for specific terms or phrases often associated with fraudulent activities.
4. Data Visualization for Clearer Insights:
Interactive Dashboards: AI-powered data visualization tools enable auditors to interact with data findings, helping to uncover hidden patterns and relationships that could indicate fraud.
Graph Analytics: This tool is effective in mapping relationships and transactions between entities, which can reveal complex fraud schemes like shell companies or insider trading.
5. Real-Time Monitoring for Immediate Detection:
Continuous Surveillance: AI systems continuously monitor financial transactions, enabling immediate detection and alerting of suspicious activities.
Automated Alert Systems: These systems can notify auditors in real-time of potential fraud, ensuring swift action can be taken.
6. Forensic Analysis Tools Powered by AI:
Digital Forensic Techniques: AI can assist in digital forensic investigations by quickly analyzing vast amounts of data to find evidence of fraud.
Scenario Analysis: AI can simulate different scenarios to determine the likelihood of fraudulent activities under various conditions.
7. Blockchain and AI for Immutable Audit Trails:
Smart Contracts: AI can analyze transactions on a blockchain to verify their legitimacy and detect any tampering or fraudulent alterations.
Audit Trail Analysis: Blockchain technology provides an immutable record of transactions, which AI can analyze for inconsistencies or irregularities.
[End of Section 3]
In this section, we explored the diverse array of AI techniques and tools that are redefining fraud detection in financial statement auditing. These innovations not only enhance the accuracy and scope of audits but also provide auditors with the means to conduct more efficient and effective investigations. The following sections will discuss the importance of the human-AI collaboration in auditing and the ethical considerations surrounding the use of AI in this domain.
Section 4: AI and Human Collaboration in Auditing
The synergy between AI capabilities and human expertise forms the cornerstone of modern auditing. This collaborative approach is particularly crucial in fraud detection, where the combination of AI’s analytical power and the auditor’s judgment and insight creates a robust and effective audit process.
Human Oversight in AI-Driven Auditing:
- Interpreting AI Findings: While AI provides valuable data-driven insights, human auditors play a critical role in interpreting these findings within the proper business and regulatory context.
- Applying Professional Judgment: The auditor’s professional skepticism and judgment are irreplaceable, especially when evaluating complex situations where AI may offer limited guidance.
- Quality Control: Human auditors must ensure the quality and accuracy of AI analyses, verifying that the AI’s conclusions are logical and based on sound data.
Ethical and Professional Considerations:
- Maintaining Auditor Independence: It’s crucial for auditors to maintain their professional independence, using AI as a tool to aid their work rather than dictate it.
- Addressing Biases in AI: Auditors must be vigilant about potential biases in AI models and ensure that these biases do not influence audit outcomes.
- Confidentiality and Data Security: As AI systems process sensitive financial data, auditors must ensure that data security and confidentiality are maintained.
- The Evolving Role of the Auditor in an AI-Enhanced Environment:
- From Data Analyst to Strategic Advisor: As AI takes over more of the data analysis tasks, auditors can focus on providing higher-level insights and strategic advice to clients.
- Continuous Learning and Adaptation: Auditors must continuously update their skills to keep pace with evolving AI technologies, understanding how these tools can be best utilized in their work.
Training and Skill Development:
- Technical Training: Auditors need training to understand how AI works, how to interpret its findings, and how to integrate these findings into the audit process.
- Soft Skills Development: Skills like critical thinking, ethical decision-making, and effective communication become even more important in an AI-enhanced auditing environment.
The Collaborative Audit Process:
- Combining AI Insights with Human Experience: The optimal audit process involves using AI to gather and analyze data, with human auditors then applying their experience and knowledge to draw conclusions.
- Leveraging AI for Enhanced Client Interactions: AI can also aid auditors in client interactions, providing them with detailed analyses and insights that can inform discussions and decision-making.
[End of Section 4]
This section highlighted the critical role of human auditors in an AI-enhanced audit environment, emphasizing the importance of collaboration, ethical considerations, and the need for continuous learning and adaptation. The next section will discuss the practical implications of integrating AI in auditing and real-world applications.
Section 5: Practical Implications and Real-World Applications
As AI reshapes the landscape of financial statement auditing, its practical implications are vast, affecting how audits are planned, executed, and evaluated. Real-world applications of AI in auditing illustrate the transformative impact of this technology.
Case Studies of AI in Auditing:
- Anomaly Detection in Large Corporations: Highlighting cases where AI systems have successfully identified fraudulent transactions in large datasets, leading to the detection of financial misstatements that traditional methods might have missed.
- AI in Forensic Auditing: Instances where AI tools have been instrumental in uncovering complex fraud schemes, such as embezzlement or money laundering, by analyzing patterns in transaction data.
Best Practices for Integrating AI in Auditing:
- Developing a Framework for AI Integration: Establishing guidelines and protocols for incorporating AI into various stages of the auditing process, ensuring consistency and reliability in AI-augmented audits.
- Training and Professional Development: Implementing continuous training programs for auditors to remain adept at using AI tools and interpreting their outputs effectively.
Preparing Auditors for an AI-Enhanced Future:
- Emphasizing Interdisciplinary Skills: Encouraging auditors to develop skills beyond traditional accounting, including data analytics, information technology, and cybersecurity, to effectively leverage AI in their work.
- Fostering a Culture of Innovation: Promoting a workplace environment that encourages exploration and adoption of AI technologies, fostering a culture of innovation and adaptability.
Challenges and Solutions in AI Adoption:
- Overcoming Skepticism and Resistance: Addressing concerns and resistance among auditing professionals regarding AI adoption, through education and demonstration of AI’s efficacy and benefits.
- Ethical AI Use and Governance: Implementing policies and frameworks to ensure ethical use of AI, including transparency, accountability, and data privacy considerations.
The Future of AI in Auditing:
- Emerging Trends and Technologies: Exploring future advancements in AI, such as enhanced machine learning algorithms and integration with other emerging technologies like blockchain.
- The Evolving Role of the Auditor: Discussing how the role of auditors will continue to evolve, with AI enabling them to focus more on strategic analysis, advisory services, and complex problem-solving.
[End of Section 5]
This section provided an overview of the practical implications and real-world applications of AI in auditing, covering best practices, training requirements, and future trends. It highlighted the need for auditors to adapt and evolve with the technology to harness its full potential effectively.
Conclusion: As we navigate the complex terrain of financial statement auditing in the AI era, it becomes evident that AI is not just a technological tool but a transformative force reshaping the auditing landscape. The integration of AI into auditing practices, especially in fraud detection, offers auditors unparalleled analytical capabilities, enabling a shift from traditional methods to more advanced, data-driven approaches.
AI’s ability to process vast quantities of data, identify patterns and anomalies, and provide predictive insights enhances the auditor’s capacity to detect fraud more efficiently and accurately. However, this technological integration doesn’t diminish the value of human judgment; rather, it complements it. The auditor’s role evolves from data analysis to interpreting AI-generated insights within the broader context of business operations, regulatory environments, and ethical considerations.
Final Thoughts: As we embrace the future of auditing in the age of AI, it’s crucial to foster a harmonious collaboration between technology and human expertise. Auditors need to adapt to this changing landscape, embracing continuous learning and skill development to stay abreast of AI advancements. Moreover, the auditing profession must address ethical considerations and data privacy concerns to harness AI’s benefits responsibly.
The future of auditing is not about replacing auditors with machines but about leveraging AI to enhance the auditor’s role. This synergy promises not only more effective and efficient audits but also a higher standard of financial integrity and transparency. As AI continues to evolve, its integration into auditing practices will become increasingly vital, marking a new chapter in the quest for accuracy, efficiency, and reliability in financial reporting